A Comprehensive Survey
Human Activity Recognition Research Paper [link]
Nowadays, the aging population is becoming one of the world’s primary concerns. It is estimated that the population aged over 65 will increase from 461 million to 2 billion by 2050. Such a substantial increase in the elderly population will have significant social and health care consequences. To monitor older adults’ physical, functional, and cognitive health in their homes, Human Activity Recognition (HAR) is emerging as a powerful tool.
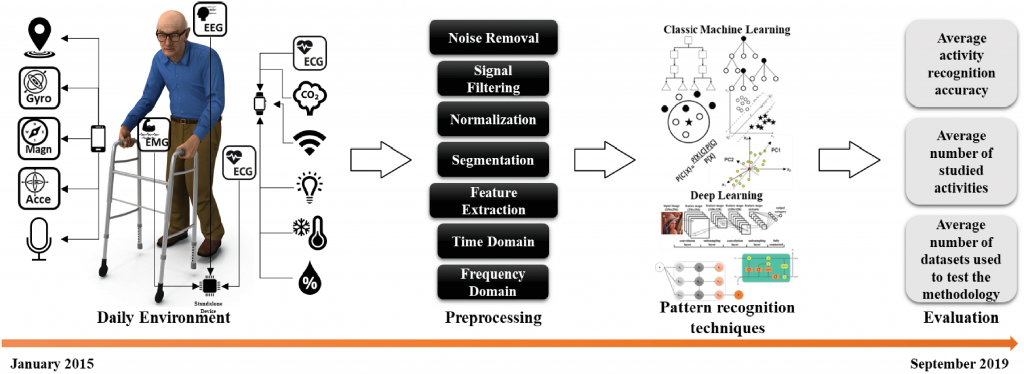
HAR’s goal is to recognize human activities of daily life (for example, walking, standing, sleeping, running, repose, watching TV, cooking, etc.) in controlled and uncontrolled settings.
i-Heal’s lab director, Dr. Parisa Rashidi, teamed up with Dr. Florenc Demrozi and Dr. Graziano Pravadelli from University of Verona, Italy and Dr. Azra Bihorac from UF Prisma-P to review the latest advancements in HAR.
This paper provides an in-depth systematic review of the state-of-the-art in HAR approaches, published from January 2015 to September 2019, based on Classic Machine Learning and Deep Learning, which make use of data collected by sensors (inertial or physiological), embedded into wearables or environment.
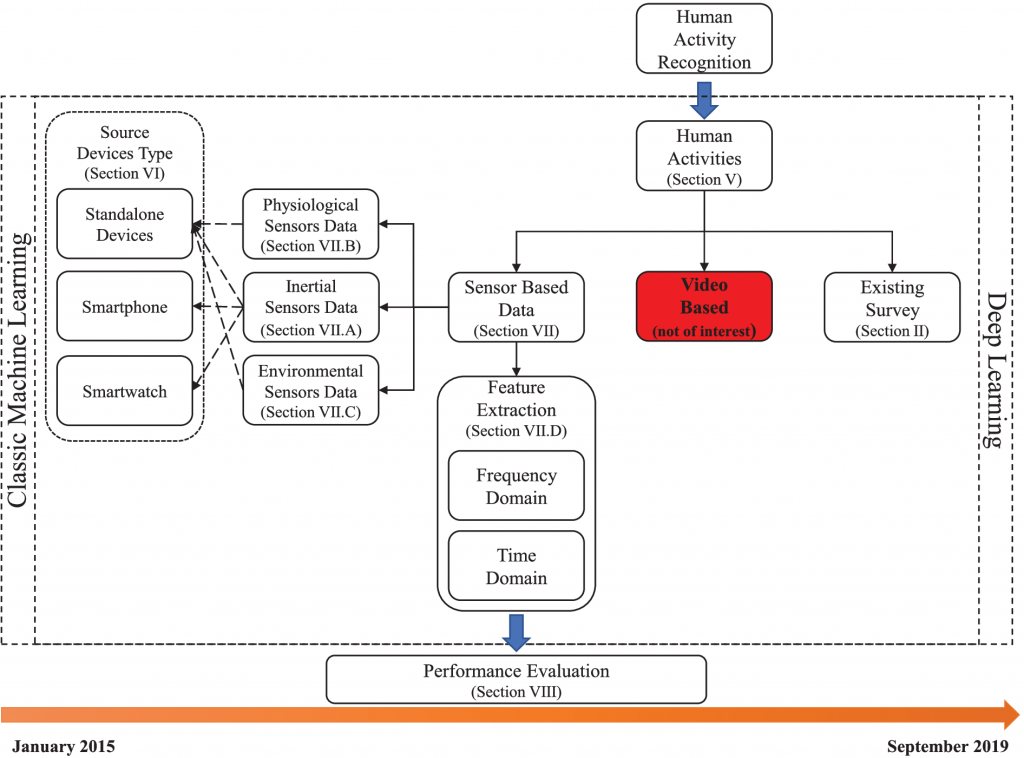
We surveyed methodologies based on sensor type, device type (smartphone, smartwatch, or standalone), preprocessing step (noise removal or feature extraction technique), and finally, their Deep Learning or Classic Machine Learning model. The results are presented in terms of a) average activity recognition accuracy, b) the average number of studied activities, and c) the average number of datasets used to test the methodology.
We presented results both from the point of view of quality (accuracy) and quantity (number of recognized activities). We concluded that HAR researchers still prefer Classic Machine Learning models, mainly because they require a smaller amount of data and less computational power than Deep Learning models. However, the Deep Learning models have shown higher capacity in recognizing many complex activities.
Future work in Human Activity Recognition applications should focus on developing more advanced generalization capabilities and recognition of more complex activities.
Read the full research paper here.
This story originally appeared on UF Biomedical Engineering.
Check out other stories on the UF AI Initiative.